When you begin data analysis, the Pandas DataFrame is an indispensable tool. It serves as the foundation of data analysis for efficiently handling tabular data. Like Excel sheets or database tables, it allows you to organize data and perform various operations with remarkable efficiency. Mastering the Pandas DataFrame greatly affects the speed and quality of your subsequent analyses. Therefore, understanding its basic structure and how to create it is essential.
You should also understand the Pandas Series, which is closely related to the Pandas DataFrame. A Series is a data structure that represents a single “column” of a DataFrame. By learning Series, you can gain a deeper grasp of how a DataFrame works.
This article provides a thorough, beginner-friendly explanation of the basic structure of the Pandas DataFrame and how to create one. By clearly outlining the differences between it and the Pandas Series, you will deepen your understanding of the DataFrame. We will also introduce the fundamental creation patterns using the pd.DataFrame
method with easy-to-follow code samples.
▶️ Be sure to consult the official documentation for DataFrame and Series as well:
- 【What You Will Learn in This Article】
- Installing Pandas
- Importing Pandas
- Pandas DataFrame Basics: Structure and Creation
- Fully Understanding the Differences Between Pandas Series and DataFrame
- ✅ Summary
【What You Will Learn in This Article】
- The basic structure and role of the Pandas DataFrame
- How to create a DataFrame from various data formats (how to use
pd.DataFrame
) - The fundamental differences between Pandas Series and DataFrame
【Related Articles】 We have separate detailed articles on how to inspect data after creating a Pandas DataFrame and how to retrieve or select specific data. Once you understand the basics of the DataFrame in this article, be sure to read the following posts to learn practical operations.
How to inspect a DataFrame:
How to extract/select data:
and more
【Personal Experience】When I first started, I didn’t understand the difference between a Series and a DataFrame and passed a 2-D list to Series, which caused an error. I realized that being aware that a Series is 1-D and a DataFrame is 2-D is the key to avoiding this initial stumbling block.
Installing Pandas
If Pandas is not installed, install it with the following command.
Importing Pandas
To use Pandas in your Python code, first import it. It is commonly imported with the alias pd
.
Pandas DataFrame Basics: Structure and Creation
What Is a Pandas DataFrame?—Your Powerful Partner in Data Analysis
The Pandas DataFrame is the most fundamental data structure for working with tabular data in Python and is the central pillar of data analysis. Like a spreadsheet or database table, it organizes data and lets you perform a wide variety of operations efficiently.
-
Read and reshape data from CSV or Excel files:
【Vol.2】Getting Started with CSV Files in Google Colab -
Extract and process only the data you need:
【Part 6】【Beginner Friendly】Basic Operations with Pandas loc to Extract Rows or Columns by Label【Illustrated】 -
Perform statistical calculations and aggregations:
【Vol.5】 Pandas info and describe for data structure & stats - Perform preprocessing for creating graphs
In other words, the DataFrame plays a role in every step of data analysis. By mastering the DataFrame, you can automate data processing that would take a long time by hand and move on to more advanced analysis. In most data-analysis projects, the DataFrame is the star of the show.
The Basic Structure of a Pandas DataFrame
A DataFrame consists of the following elements.
- Data: The values in the table. They can hold various data types (numbers, strings, etc.).
- Column names (Columns): The labels for each column. Each column can be thought of as a one-dimensional data structure called a “Series.”
- Index: The labels for each row. By default it is a sequence starting at 0, but you can set any values you like.
The following illustration shows the structure of a Pandas DataFrame.
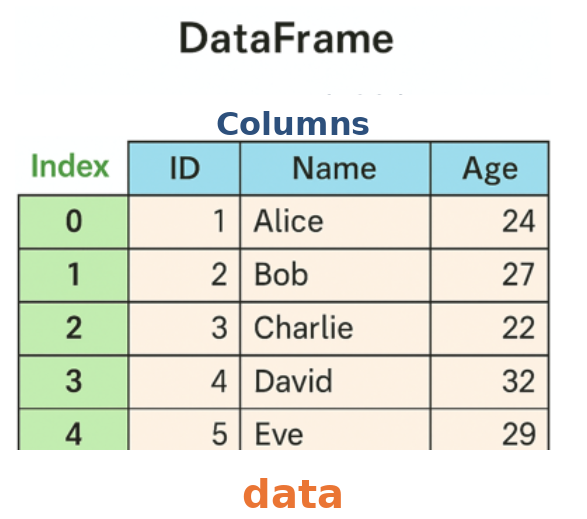
Creating a Pandas DataFrame
You can create a Pandas DataFrame from various data formats using the pd.DataFrame()
method. Below are the main creation patterns commonly used in data analysis.
Create from a List of Lists
A common approach is to use a “list of lists” in Python (a list that contains other lists). In this structure, each element of the outer list becomes a row, and the elements of the inner lists become the values of each column. It is typical to specify the column names with the columns
argument.
DataFrame created from list of lists: ID Name Age 0 1 Alice 24 1 2 Bob 27 2 3 Charlie 22 3 4 David 32 4 5 Eve 29
Create from a Dictionary
You can also pass a Python dictionary to pd.DataFrame()
. In this case, the dictionary keys become the column names of the DataFrame and the values (lists or NumPy arrays, etc.) become the column data. For many cases, this method is an intuitive way to create a DataFrame.
DataFrame created from dictionary: ID Name Age 0 1 Alice 24 1 2 Bob 27 2 3 Charlie 22 3 4 David 32 4 5 Eve 29
Create from a NumPy Array
If your data already exists as a NumPy array, you can pass it directly to pd.DataFrame()
to generate a DataFrame. As with the list-of-lists approach, you can specify the columns
and index
arguments.
DataFrame created from NumPy array: ID Name Age 0 1 Alice 24 1 2 Bob 27 2 3 Charlie 22
Creating and Modifying with a Specified Index
The Pandas DataFrame allows you to freely set or change the row labels, called the index.
The index is a label that uniquely identifies each row in a DataFrame. By default, an integer sequence starting at 0 is assigned automatically, but you can set meaningful values—such as dates or IDs—depending on your data. The index plays an important role when efficiently selecting specific rows or when joining multiple DataFrames.
You can set a particular column as the index by using the set_index()
method.
DataFrame before setting the index: ID Name Age 0 1 Alice 24 1 2 Bob 27 2 3 Charlie 22 3 4 David 32 4 5 Eve 29 'ID'DataFrame after setting the 'ID' column as the index: Name Age ID 1 Alice 24 2 Bob 27 3 Charlie 22 4 David 32 5 Eve 29
Other Creation Methods (Reading from Files, etc.)
Besides the approaches above, you can create a Pandas DataFrame in the following ways.
- Read external files such as CSV or Excel (
pd.read_csv()
,pd.read_excel()
, etc.) - Create from a dictionary of Pandas Series
For details on reading CSV files, see the article 【Part 2】Google Colab and Drive: Easy CSV Loading and Saving | Beginner Drive Integration Guide.
【Cautions When Creating a DataFrame】
When creating a DataFrame from a list of lists, pay attention to the structure of your data—for example, you will get an error if the inner lists do not all have the same number of elements.
Fully Understanding the Differences Between Pandas Series and DataFrame
What Is a Pandas Series?—Its Role and Relation to DataFrame
A Pandas Series is a one-dimensional, labeled data structure. It resembles a list or a NumPy array, but differs in that it carries an index.
It is easier to grasp its role if you think of a Pandas Series as a single column that makes up a Pandas DataFrame.
【When Should You Use Series?】 A Series is useful, for example, when you want to pull out just one column from a DataFrame for analysis. Of course, you can also use it on its own when you need to handle one-dimensional data.
Extracting a Series from a DataFrame
Selecting a specific column from the DataFrame created in the previous section yields a Pandas Series. Let’s confirm this relationship with code.
Original DataFrame: ID Name Age 0 1 Alice 24 1 2 Bob 27 2 3 Charlie 22 3 4 David 32 4 5 Eve 29 'Name'column (retrieved as Series): 0 Alice 1 Bob 2 Charlie 3 David 4 Eve Name: Name, dtype: object Type of retrieved data: <class 'pandas.core.series.Series'>
Basic Ways to Create a Series
You can also create a Pandas Series from various data formats using the pd.Series()
method. To understand that a DataFrame column is in fact a Series, let’s briefly look at the basic creation patterns. We will cover the details in another article.
Series created from a list: 0 10 1 20 2 30 3 40 dtype: int64 Series created from a dictionary: a 10 b 20 c 30 d 40 dtype: int64
Key Differences Between Series and DataFrame (Structure, Dimensionality, Usability)
When learning Pandas, it is crucial to clearly understand the differences between Series and DataFrame. The main distinctions are as follows.
Dimensionality:
- Series: One-dimensional data structure
- DataFrame: Two-dimensional data structure (rows and columns)
Structure:
- Series: Data are arranged in a single column, and each item has an index (label).
- DataFrame: A table format with rows and columns; each column has a name and each row has an index.
-
Usability:
-
There are differences in the code you write to select and manipulate data. For example, from a DataFrame you can select a specific column (Series) by its name or use
.loc
and.iloc
to select rows or individual elements. From a Series, on the other hand, you select elements by index label or position. -
Another important point is that using
[]
returns a Series (1-D), while using[[]]
returns a DataFrame (2-D). - Detailed explanations of these operations can be found in the articles below:
Understanding these differences makes it clear when you should work with a Series versus a DataFrame and lets you write code more efficiently.
-
There are differences in the code you write to select and manipulate data. For example, from a DataFrame you can select a specific column (Series) by its name or use
The following figure visually demonstrates this structural difference.
✅ Summary
This article thoroughly explained the basic structure of the Pandas DataFrame, the cornerstone of Pandas-based data analysis, and the fundamental ways to create one from lists and dictionaries using the pd.DataFrame()
method.
We also deepened our understanding of the Pandas Series—an element that makes up a DataFrame—and the fundamental differences between Series and DataFrame in terms of dimensionality, structure, and usability.
By solidifying these Pandas fundamentals, you are now ready to progress through the various stages of data analysis.
To truly master the Pandas DataFrame, knowing how to create it is only the first step—the subsequent data manipulation is equally important. Be sure to check the upcoming articles to further level up your data-analysis skills with the Pandas DataFrame.
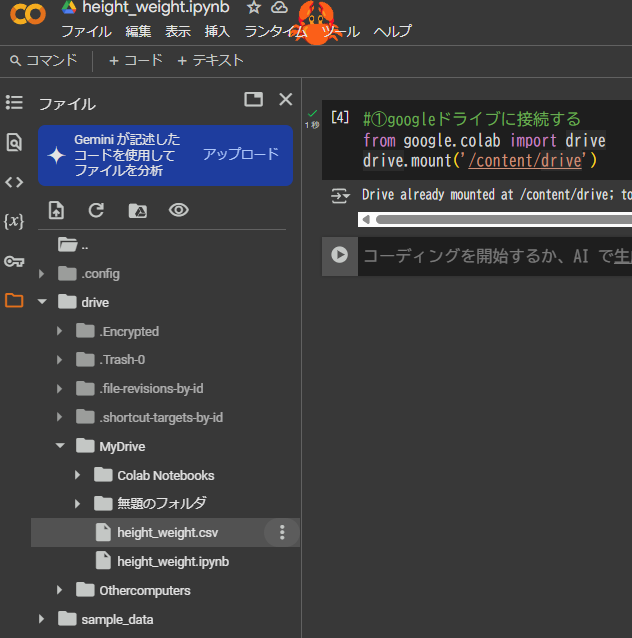
コメント